Introduction to Deep Learning
Deep learning is a sub-technique of machine learning and is primarily concerned with algorithms. It enables computer systems to mimic how humans behave in specific situations, thereby reducing human intervention in operations and facilitating full automation.
Deep learning makes possible the conception and execution of impressive feats such as automatic parking in cars and other previously impossible feats. Through deep learning, models can achieve human-level performance, or sometimes even exceed it.
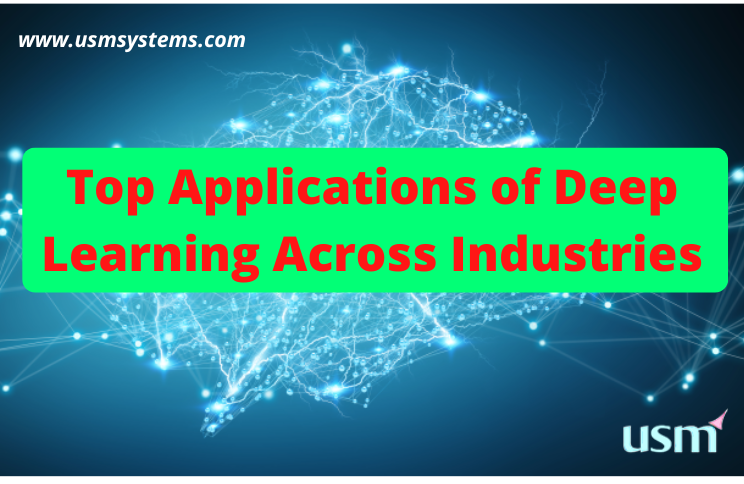
Applications of Deep Learning in Natural Language Processing
Teaching machines the semantics, syntax, expressions, tonal nuances, etc. of a language is not an easy task, all of which require humans years and years of interaction and exposure to different social settings to understand and pick up. Importance of Natural Language Processing trains machines through deep learning and generating appropriate responses for each scenario.
Examples of best deep learning applications in NLP include: document summarization, text categorization, language modeling, question answering, Twitter analysis, sentiment analysis, etc. in the legal field.
Deep Learning Applications in Ecology
As a rapidly developing extension of machine learning, deep learning has yet to receive the attention it deserves in ecology. However, its applications have been found to support the fight against global issues such as climate change, biodiversity loss, increasing demand for ecosystem services, environmental assessments, etc. Easy availability of data and improved computing power have made quantitative approaches possible.
Examples of deep learning in ecology include: aquaculture farming, coral reef protection, identification and analysis of animal populations, management and conservation, etc.
Deep Learning Applications in the Military
Armed with AI and deep learning, military systems can efficiently handle large amounts of data and are a vital part of modern warfare due to efficient computing and decision-making capabilities. In times of immediate threats, deep learning solutions streamline analysis and facilitate quick decision-making through critical insights.
Deep learning also enables intelligence gathering for better prediction of battle scenarios and faster air or ground analysis. It also helps in understanding enemy behavior and communication.
Examples of deep learning in the military include: warfare platforms, cybersecurity, logistics and transportation, target identification, battlefield healthcare, combat simulation and training, threat monitoring, etc.
Applications of Deep Learning in Virtual Assistance
Virtual assistants, Siri, Alexa, Cortana, Google Assistant, etc. are all very popular applications of deep learning. Each interaction with them allows them to learn more about your voice and, therefore, give you the experience of a secondary human interaction.
Deep learning helps these AI Voice Assistant Apps learn about your preferences and provide you with a better and more personalized experience by offering suggestions from favorite hang-outs to music.
Examples of deep learning applications in virtual assistants include: translating speech to text, note-making, appointment booking and management, automatically responding to specific calls, text generation, document summaries, composing and sending emails, etc.
Deep Learning Applications in Visual Recognition
Deep learning allows images to be efficiently sorted by locations detected in them, facial recognition and events, dates and many other possible categories. Visual recognition systems consist of several basic-advanced layers. Large-scale applications in this field through Deep Neural Networks are fueling growth in digital media management and widely observing implementations of Python, Tensor Flow, and Convolutional Neural Networks.
Examples of deep learning in visual recognition: stock photography websites, video websites, automated image organization, visual search for better product discovery, image classification for websites with large visual databases, etc.
Deep Learning Applications in Big Data
Deep learning enables the analysis of enormous unsupervised datasets that prove to be a valuable tool for big data analytics. It can extract complex patterns from these huge volumes of data, data tagging, semantic indexing, quick data retrieval, and sorting discriminative tasks.
Advanced algorithms based on deep learning techniques are critical for accuracy and efficiency during real-time data processing.
Examples of deep learning in big data: Automated extraction of complex data, from massive amounts of unsupervised data, simulations, social media, classification, prediction, etc.
Deep Learning Applications in Robotics
Deep learning is increasingly used to build robots to perform human-like tasks. Robots powered by deep learning use real-time updates to sense obstacles in their path and instantly pre-plan their journey. It can be used to carry goods in hospitals, factories, warehouses, inventory management, manufacturing products etc.
Medical imaging, genome analysis, new drug discovery, early and accurate diagnosis of life-threatening illnesses, augmented clinicians, standardization of pathology results and treatment regimens, forecasting of future health risks, etc. are a few examples of how deep learning is being used in healthcare.
Deep Learning Applications in Image colorization
Image colorization using deep learning has made significant progress. Image colorization takes an input of a grayscale image and then produces an output of a color image. Chromogen is an example of a picture colorization model. A generative network modeled on an adversarial model learns to color by incorporating perceptual and semantic understanding of both class distributions and color.
In the entertainment sector, deep video analysis, audio/video syncing and testing, transcriptions, tagging, content editing, auto-content creation, cameras that can analyze body language for movies, etc. are all examples of application areas for deep learning.
Deep Learning Applications in News Aggregation and Fake News Detection
Deep learning allows you to customize news based on reader personality. You can aggregate and filter news information according to social, geographic and economic parameters and the personal preferences of the reader. Neural networks help develop classifiers that can spot fake and biased news and remove it from your feed. They also alert you to potential privacy violations.
Examples of deep learning applications in fraud detection include risk profile development, e-commerce, identity theft prevention, payment fraud prevention, fraud detection in e-commerce, email risk scoring, etc.
The end
Deep learning has found its importance in almost every business. It is used in e-commerce, healthcare, advertising, manufacturing, entertainment and many other industries.
Deep learning has revolutionized our lives by making things easier. Do you have any questions regarding this article on Deep Learning Applications? Then, please leave your queries/inputs in the comments section. We will be glad to help you. At USM, we provide advanced Applications of deep learning in Newyork that help you build intelligent artificial neural network models to learn and make intelligent decisions automatically.
Comments